Panel Discussion
Learning versus Understanding: is AI a Definitive Game Changer in Science?
Tuesday, July 6, 2021
16:30 - 17:30 CEST
Virtual Room: Ella Maillart
Full recording available here.
Artificial intelligence and machine learning have rapidly become a must across the breadth of scientific research activities, as well as opening new directions in emerging computational disciplines. Can this be attributed to a failure of the traditional scientific methodology or is it due to a synergy of rapid HPC development with a data deluge that cannot be exploited otherwise?
AI and ML can automate the scientific method (hypothesis generation, experiment design, data collection, analysis and inference). In particular there has been a lot of recent activity in hypothesis generation with generative models/causal learning/knowledge representation. AI may “augment” humans through efficient collaboration to achieve true collective intelligence, overcoming human flaws (narrow framing of a problem, personal bias, ego, pride and investment in “sunken cost,” and so on). How can we identify the areas of research with greatest opportunities to exploit this potential and ensure an optimum combination of talent and tools to yield an efficient accumulation of wisdom?
Can ML/DL fully replace a knowledge-based analytical or numerical solution of a physical problem through a “black-box”? Is that more efficient and how can trust be maintained?
The training of AI systems has very high costs in terms of computing resources, energy cost and related CO2 production. Do the benefits outweigh these costs? Is it sustainable and what might a green AI/ML look like?
Can scientists combine traditional scientific and computational methodologies with AI to achieve the best of both worlds?
Moderator
Maria Girone (CERN, Switzerland)
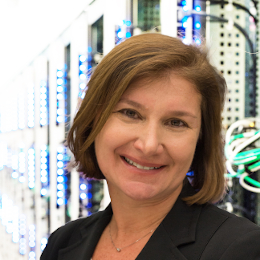
Maria Girone has a PhD in particle physics. She also has extensive knowledge in computing for high-energy physics, having worked in scientific computing since 2002. Maria was an early developer of services and tools for the Worldwide LHC Computing Grid and was founder of the WLCG operations coordination team. From 2014 to 2015, Maria was the software and computing coordinator for the CMS experiment. Maria joined CERN openlab as CTO in 2015. She is managing the overall technical strategy of CERN openlab plans towards R&D in computing architectures, HPC and AI, and promoting opportunities for collaboration between science and industry. Since July 2020, Maria coordinates for CERN the HPC Collaboration with SKA, GÈANT, and PRACE to tackle challenges related to the use of HPC for large data-intensive science.
Panelists
Trilce Estrada (University of New Mexico, US)
François Fleuret (University of Geneva, Switzerland)
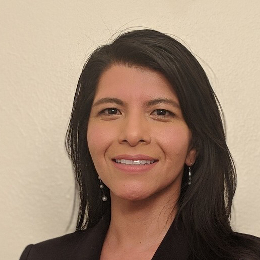
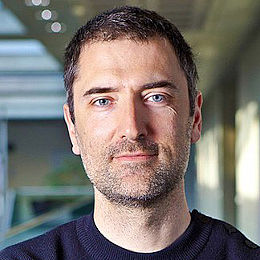
Trilce Estrada is an associate professor in the department of Computer Science at the University of New México and the director of the Data Science Laboratory. Her research interests span the intersection of Machine Learning, High Performance Computing, Big Data, and their applications to interdisciplinary problems in science and medicine. Estrada received an NSF CAREER award for her work on in-situ analysis and distributed machine learning. In 2019 she was named the ACM SIGHPC Emerging Woman Leader in Technical Computing. Currently she is the Big Data aspect lead for the NSF-TCPP national curriculum development initiative and PI faculty advisor of the New México’s Critical Technology Studies Program. Estrada obtained a PhD in computer science from the University of Delaware, a M.S in Computer science from INAOE, Mexico and a B.S in computer systems from The University of Guadalajara, Mexico.
François Fleuret is Full Professor and head of the Machine Learning group in the department of Computer Science at the University of Geneva, Adjunct Professor in the School of Engineering of the École Polytechnique Fédérale de Lausanne, and External Research Fellow at the Idiap research institute. He got a PhD in Mathematics from INRIA and the University of Paris VI in 2000, and an Habilitation degree in Mathematics from the University of Paris XIII in 2006. He is the inventor of several patents in the field of machine learning, and co-founder of Neural Concept SA, a company specializing in the development and commercialization of deep learning solutions for engineering design. His main research interest is machine learning, with a particular focus on computational aspects and sample efficiency.
Nikos Konstantinidis (UCL, UK)
Jibo Sanyal (ORNL, US)
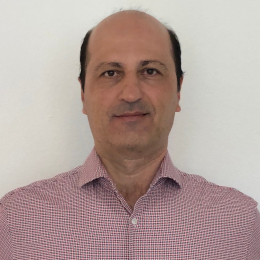
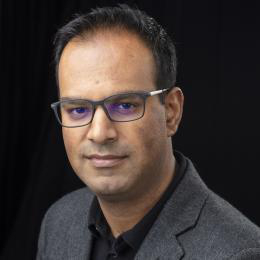
Nikos Konstantinidis is Professor of Experimental Particle Physics at UCL. He has been involved in the ATLAS experiment at CERN’s LHC for the past two decades and is an expert in real time event filtering (triggering) and pattern recognition for the reconstruction of charged particle trajectories (tracking), two of the most data- and CPU-intensive challenges for the LHC Experiments. He was the UK National Contact Physicist for ATLAS in 2016-18, and since 2017 he has been leading the STFC Centre for Doctoral Training in Data Intensive Science (DIS) at UCL, which aims to foster cross-disciplinary interactions and industry-academia knowledge exchange in novel DIS techniques.
Jibonananda (Jibo) Sanyal serves as the Group Leader for Oak Ridge National Laboratory’s Computational Urban Sciences research group. His work falls at the intersection of high performance computing, extreme scale data and analytics, modeling and simulation, visualization, scalable machine learning, and sensors and controls for building both research and operational systems focused on complex urban systems at local, regional, as well as national scales. He has extensive experience applying these techniques towards developing insights and solutions for urban dynamics, energy and smart-grid applications, situational awareness tools, weather and flood impacts, emergency response and resiliency, building energy modeling, transportation and mobility, and cyber-physical control. He is an IEEE Senior Member and a 2017 Knoxville’s 40 under 40 honoree.